A Challenging Path to Building Predictive Analytics into IIoT Deployments
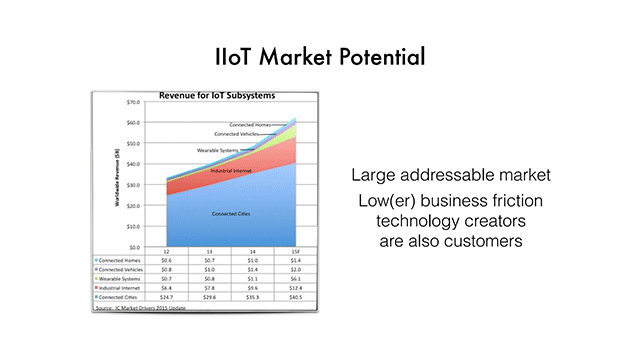
Huge IIoT potential
Why is the Industrial Internet of Things different from other types of modern deployments (or is it)? Dr. Venu Vasudevan, a Chicago-based IoT consultant, who also serves as an adjunct professor at Rice University, addressed this question at a recent Predix meetup in Chicago that was co-organized by Altoros.
Venu provided some market research by IC Market Drivers showing the IIoT as a $12-billion market in 2015, with a growth rate that experiences a doubling every three years or so. (This tracks well with anticipated overall data growth rates from the Cisco research.)
It’s about transformation, not just data
However, the heart of this discussion focused on transformation rather than simply growth. “An enterprise’s data needs to move from (mere) reliability to optimization,” he said. “It must migrate from being descriptive and reactive to predictive.”
He cited a survey from an IoT pioneer Parstream (a German-based company that Cisco acquired in November 2015) that states “IoT is not delivering its full potential because of data challenges. (Whereas) 86% of enterprises say IoT data is important, only 8% of them are capturing it in a timely fashion.” The survey also found out that 70% of respondents said improved IoT data collection would improve their decision making.
“Only 8% of enterprises are capturing IoT data in a timely fashion.” —Dr. Venu Vasudevan
As much of newly created IIoT data is coming from network edges (along factory floors, etc.), it’s critical to focus on optimal ways to handle this data, Venu pointed out. He outlined a transition from the popular conception of “slow” data lakes to “fast data streams,” mentioning an acceleration of 30 to 100 times.
The need for real-time architecture
Venu espoused the use of (admittedly complex) lambda architecture, involving the use of Apache Spark, as the path to success in deploying and successfully managing these new, fast data streams. He further recommended edge filtering systems as a way to get a good grip on useful data from these streams early in the process, as abstracted and illustrated in the following diagram:
Massive IIoT data production and handling systems imply the idea of “machine learning at scale,” he noted, as companies work to establish the procedures that will produce the clean data that’s needed for predictive analytics to meet its potential.
Cleansing the data
He noted the significant differences in gathering and using consumer data versus IoT data—consumer data capture is hard, IoT (and by extension, IIoT) easy; yet consumer-data sanitizing is only moderately difficult while IoT-data sanitization is difficult, and modeling of the two types of data is easy and difficult, respectively.
Intelligent integration
The resultant “insight-data gap” with IoT data behooves the use of a two-tier IT structure, in which a machine learning (ML) approach to “wrangling” of IoT data is present.
Nevertheless, an intelligent integration to a target model is still needed.
Cloudy, edgy forecast
In conclusion he abstracted the present and future of the IoT as follows:
Present: Cloudy
- Embrace: leverage cutting edge cloud and ML services.
- Extend: adapt to IIoT business processes.
Future: Edgy
- Hyper-decentralized intelligence and data.
- Systems that understand “normal” and “deviation”.
- Predictive systems that have both response velocity and
depth of insight.
Where are we on the path?
Venu’s presentation and synopsis struck a few deep chords in me:
It was another demonstration that shows how early we are in the Predix era. Altoros has now sponsored more than a dozen of Predix meetups, yet we remain in sort of a "pre-Predix" era, in which developers and enterprises need to figure out what they’re doing and what they want to achieve before they can even think of building a PoC, pilot project, or use case for Predix.
The sanitizing (or cleansing) raw data is an enormous issue. Venu outlined his two-tier approach, something that will be complex, developing, and probably tedious. Existing problems of finding the gold in the ore are only magnified in an IIoT installation that features a rapid data stream instead of a placid data lake.
Venu’s closing remarks about understanding the difference between "normal" and "deviation" is similar to many conversations I’ve had recently about turning "anomalies" into "patterns." With a massive, ongoing data flow—an archetype being the data that GE collects from its jet engines—things that in the past that were unlikely therefore aberrational reveal themselves to be uncommon but predictable.
This strikes me as a big thought, as we contemplate all of the new insights that the era of the IIoT and big data can reveal. Will it turn out that there’s nothing truly aberrational, that in fact all data has some order to it within the famed Lorenz Attractor (i.e., "butterfly") within chaos theory?
"Modeling consumer data is easy, modeling IIoT data is difficult." —Dr. Venu Vasudevan
Related slides
Related reading
- Going Loco with GE Predix and Siemens Analytics
- GE Predix and the DDS Standard Transform Healthcare, Control Robots