What Is ETL (Extract, Transform, and Load)?
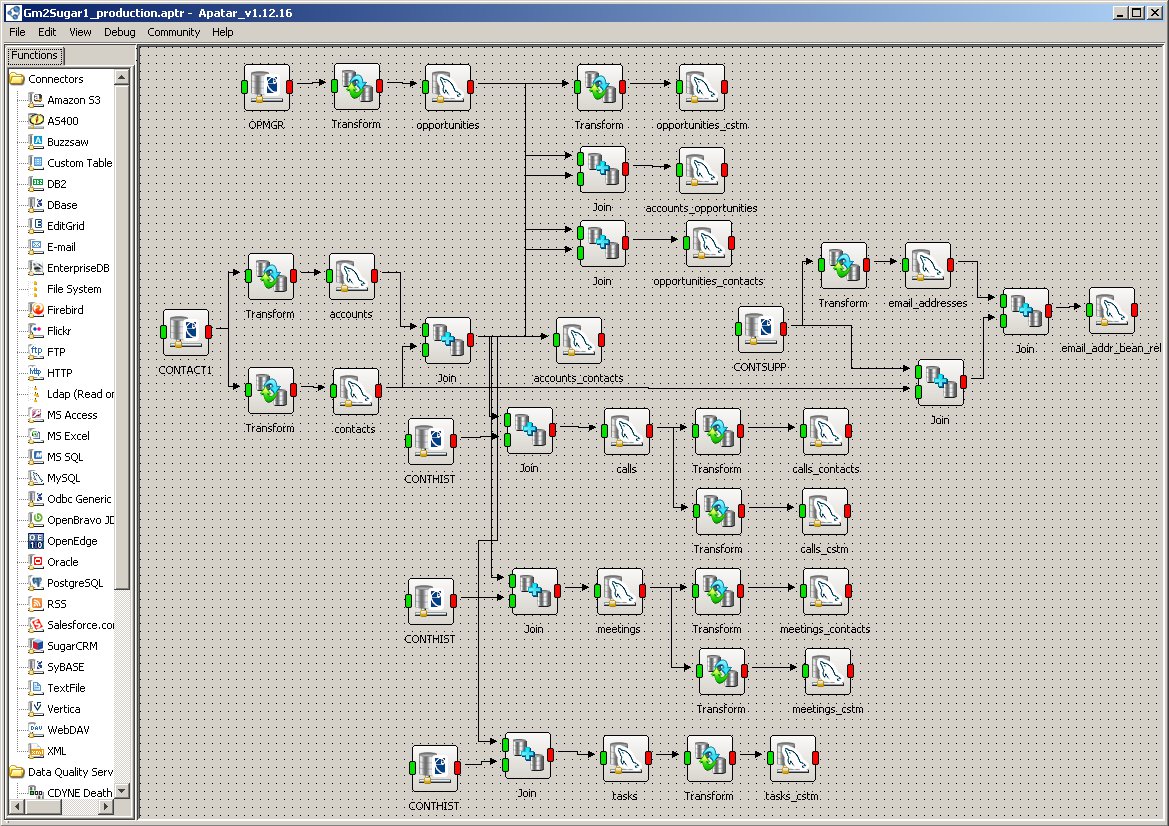
Introduction
In brief, ETL processes are used to extract data from various sources, transform it by cleaning and integrating, and finally load it into data warehouses, databases, or corporate systems. The data you get in the end is clean, well-structured, systematic, and ready to use. It sounds quite easy, but in reality there’s much more to it. There’s a catch to each step.
1. Extract
During the first step, data extraction, the challenge you are dealing with is the bulk of data from different sources. It might be different departments, databases, formats, reporting systems, etc. This scattered data needs to somehow be captured and moved into the staging database.
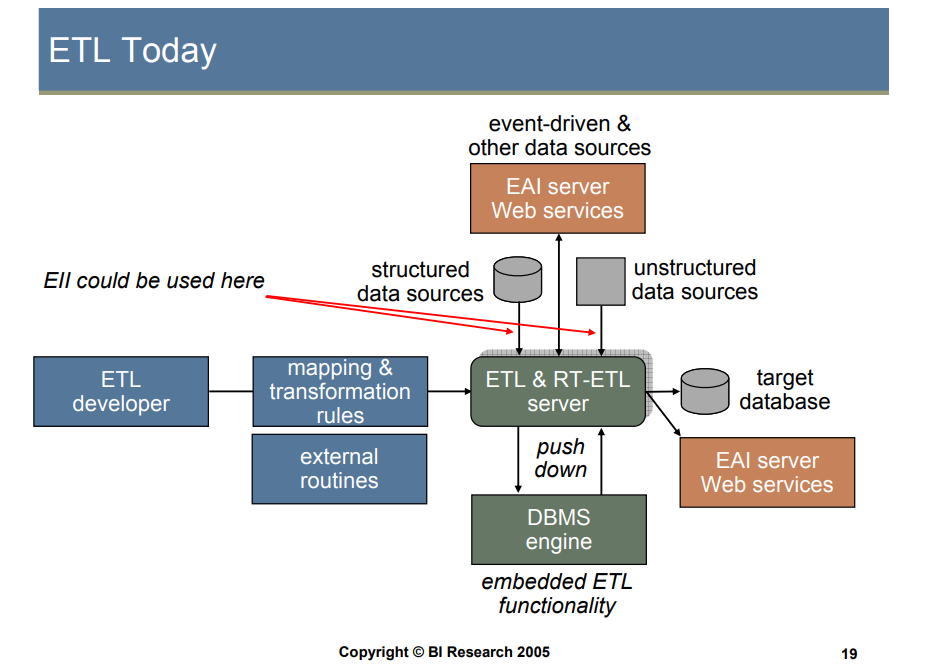
In order to work with heterogeneous data structures, an ETL tool should:
- Work with a variety of formats and databases
- Convert flat files, unstructured data
- Define mappings and transformation rules, storing them independently from the actual implementations
Constructing connections to each of the source technologies may become challenging. So, prebuilt mappings and connectors provided by ETL tools facilitate this effort greatly.
2. Transform
Next, data transformation—this step is the most complicated one. It can be broken into four separate steps under the umbrella of transformation:
- data verification—comparing the extracted information with the data warehouse’s quality standards. In case data doesn’t meet the outlined standards, it either gets rejected, or held to be reviewed by the administrator.
- data cleansing—the data left from the previous step is made more precise. (The techniques this stage includes are so many that they deserve a separate post.)
- data mapping—merging data from different sources into a single interface, structuring it into columns and tying it together logically.
- data consolidation (or aggregation)—summarizing data from the previous step and performing overall calculations to provide the user with a more complete picture.
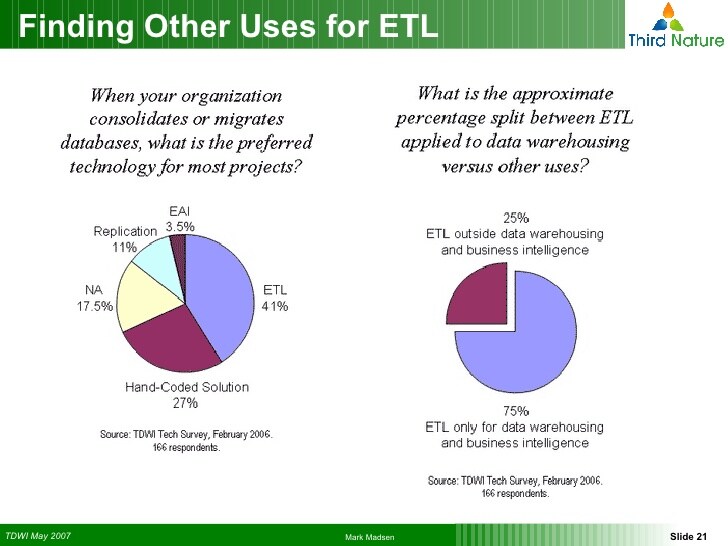
3. Load
Finally, loading. This step simply uploads the data organized during transformation into a warehouse.
During this whole process, the one thing to be careful of is losing data. ETL process does not presuppose changing the initial data, it should only make it better, cleaner, more correct and organized.
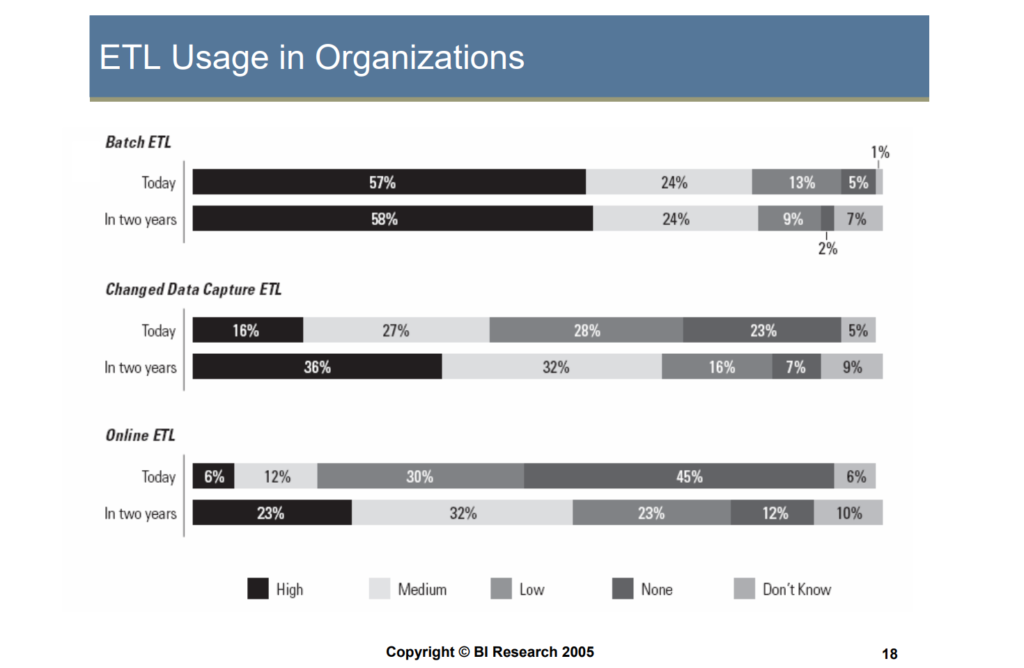
Finally, ETL can be utilized across numerous scenarios and needs. This can be either a one-time activity, or an ongoing process. You can implement either batch ETL jobs, or deal with real-time data—and so on and so forth.
p.s. If you need an ETL tool, explore Apatar.
Further reading
- Top Data Integration Challenges: Meet DQ, CDI, EAI, DW, and BI
- ETL vs. ESB from Apatar’s Point of View
- Reducing ETL and Data Integration Costs by 80% with Open Source