Whom to Blame for no Success in Data Integration?
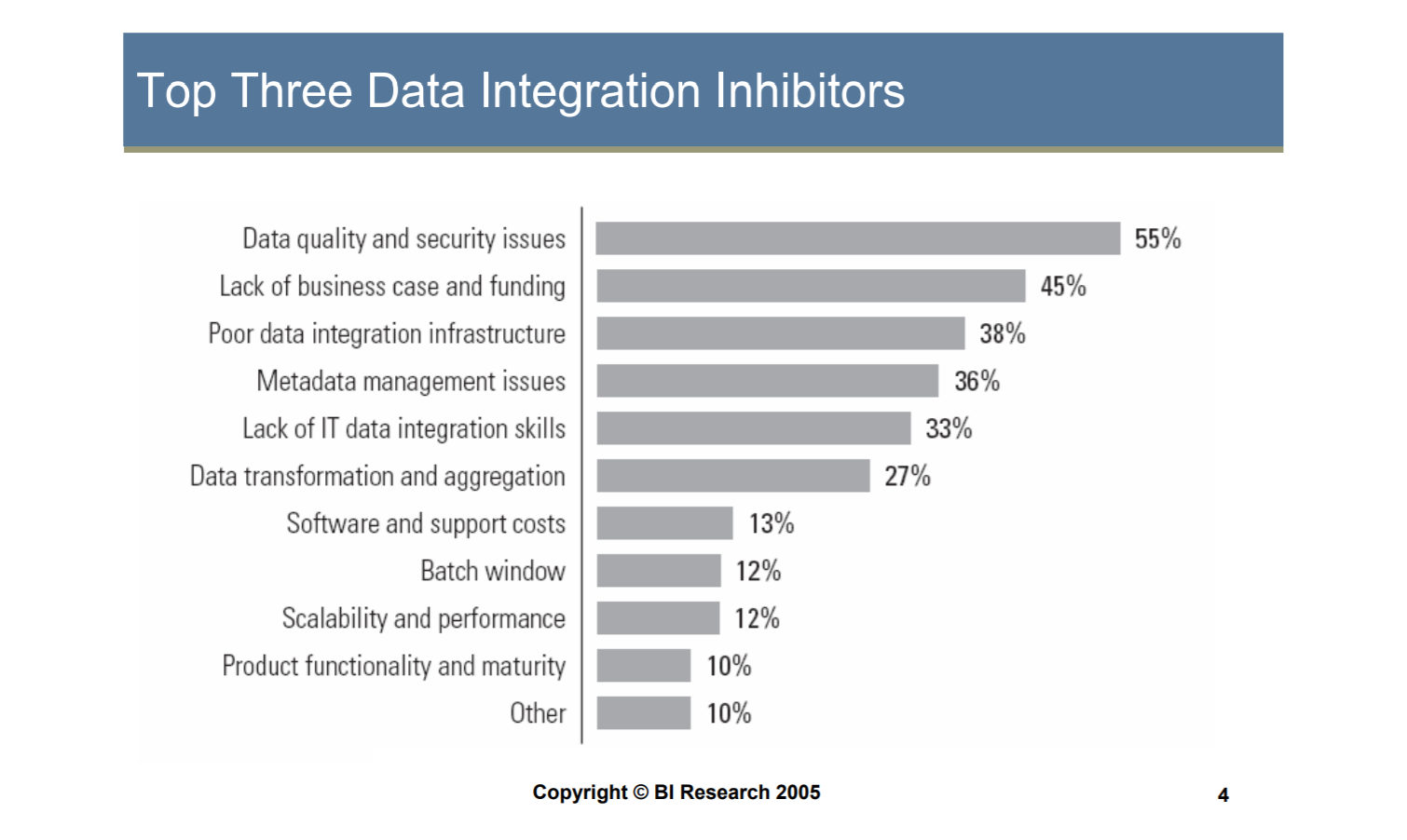
A growing demand for data integration
Data integration software market shows no sign of slowing. In February 2009, Gartner predicted the 17% annual growth for the data integration software market over the next four years. What makes data integration professionals expect a high demand for their services?
Well, now it is obvious that data is not just information stored for the sake of compliance, but that it holds a great value to corporate decision-makers. This understanding is not simply about providing a basis for sales and marketing, but also about accurate and timely data being the foundation for business performance management.
Today, data integration has stretched far beyond basic extraction, transformation, and loading (ETL). The demand for experts and software capable to cope with data integration challenges—such as cleansing data and improving the data content, mapping data elements to some standard or common value, transforming data that does not meet common expected rules, and coping with the situations when these rules fail—is growing as data projects become larger.
In this process, ETL adoption is regarded as a prelude to the implementation of corporate-wide, standard business intelligence software.
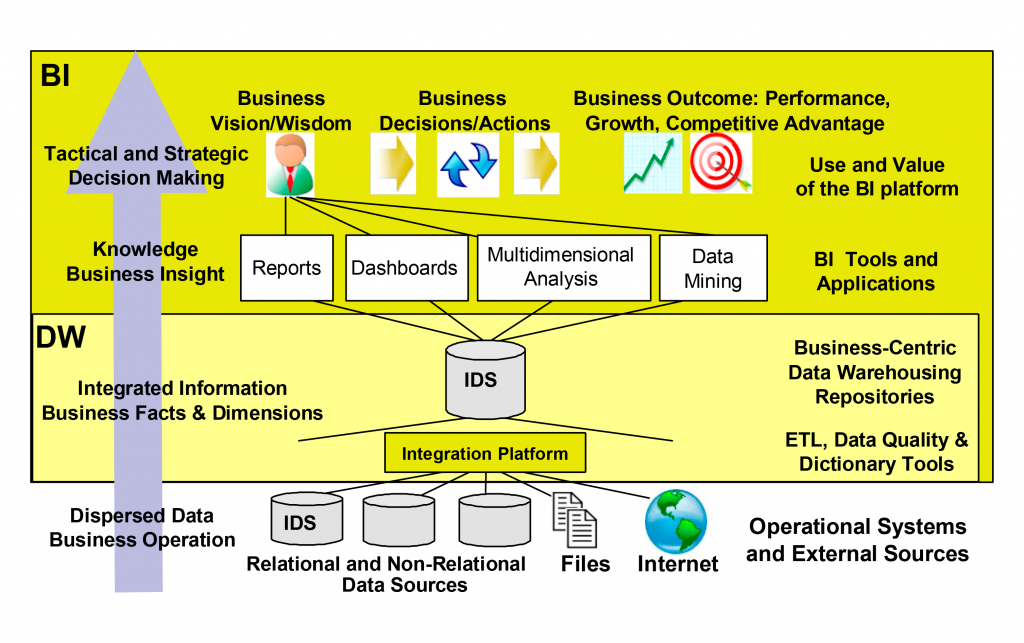
The sources of data integration issues
At the same time, very often, we hear about the failures and challenges that companies face when starting data integration initiatives. So, what are the sources of these issues?
According to an interesting article by data management analyst Noreen Kendle I found last fall, the typical tools/technology approach of merging data from various database systems doesn’t work due to a number of things:
Methodology. Tools simply do their job on merging based on data field names or content. Assuming field names may be misleading, you can imagine the mess that may come out of it.
Dimensions of time. Time bring changes. This is true about data as well: data meaning changes over time, so two items that seem identical, but represented by different time mark may mean rather different things.
Capturing data relationships. The full meaning of data may be lost if the data is viewed individually, with no reference to its relations with other data. There should be relative meaning of data items preserved somehow in order to preserve data integrity.
So, the better the data’s quality and structure/relationships are, the better tools/technology approach to data integration works. However, all this is insufficient without understanding the business meaning of data—i.e., the data should be mapped to a business data model, and only then it is ready for integration.
New challenges ahead
As the amount of data grows exponentially, the requirements to ETL systems get more complex. Today, ETL providers face some new challenges along with traditional data integration issues:
Scalability. ETL systems need to be able to process large volumes of data that intend to keep growing. Moreover, today’s business reality requires getting more data in less time. So, scalable ETL is a must.
Interoperability. A large company’s IT system comprises multiple disparate sources of business-critical data, such as databases, CRM systems, etc. These days, ETL tool should have connectivity to all those systems. Moreover, data integration between all data sources often requires complex transformations to make the data fit the formats common for this or that system.
Real-time data integration. This requirement is being heard more and more often. Business users want access to real-time information to make better decisions. The need for real-time data demands from ETL systems the ability to process extract-transform-load operations and gather all the data in a standard, homogeneous environment in a really short period of time.
Finally, the cloud. As cloud offerings get mature and provide some beneficial solutions (especially for small and mid-sized business), companies decide to move parts of their applications to the cloud. Providing smooth connectivity to cloud systems is a today’s ETL challenge, as well.
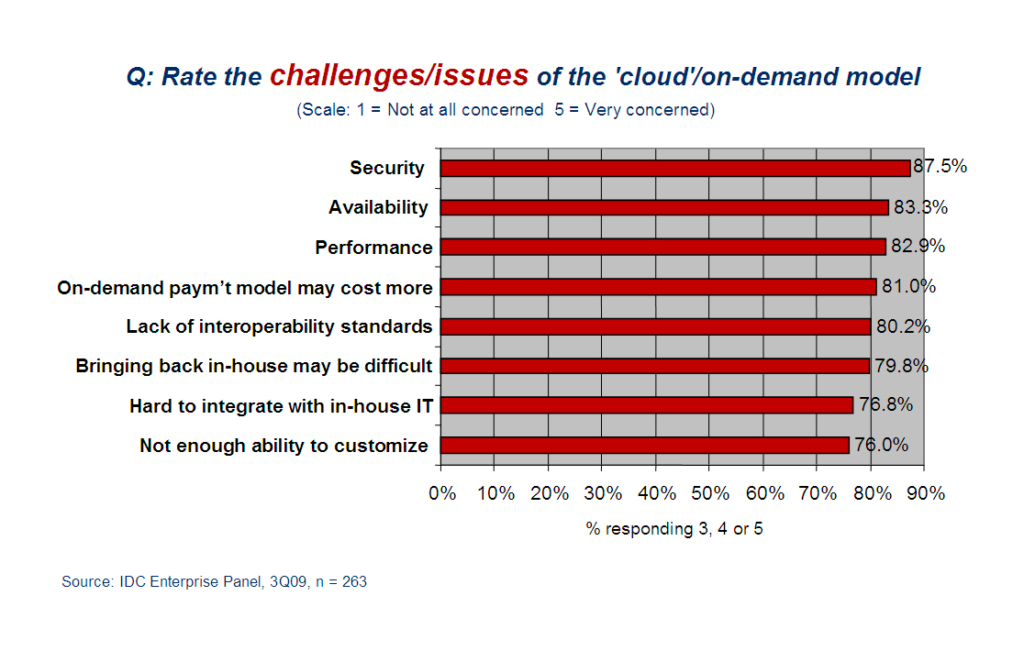
However, a lot of organizations still regard data integration as mostly a technological process, not taking into account how it impacts organization’s long-term plans and the success (or failure) of business. Therefore, the success of data integration may require a shift in a mindset, too.
Further reading
- Gartner Names Five Approaches to Successful Data Integration
- ETL Deployment: Ensuring Right Expectations
- Corporate Analysis and Business Intelligence Lack Data Quality