Avoiding Common Data Quality Management Mistakes
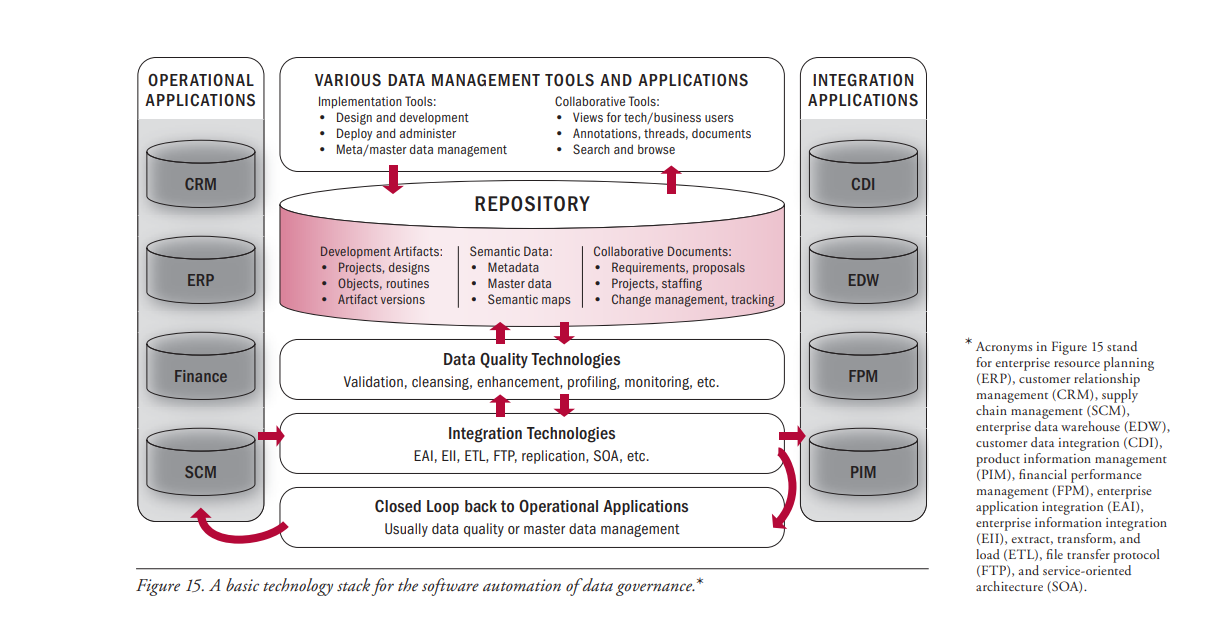
(Featured image credit: TDWI)
Major issues
Due to the size and complexity of an organization, data quality management can be quite complicated and, sometimes, may turn (and very often it does) into a nightmare. There are three most common mistakes that companies make when starting a data quality management project:
- Hoping for a “magic tool.” It means that too often organizations believe a packaged solution can “fix” noncompliant data though in the first place they should care about eliminating the introduction of bad data. Although data quality tools are the critical components of a data quality program, one must first question the motivation for purchasing a tool, then the process itself, and consider the improvement potential in terms of contributing to the effectiveness of the program.
- Lack of the right expertise. The success in developing a data quality management program depends on having both business and technical expertise but, in fact, lots of organizations disregard this crucial moment, being sure that as soon as a data quality program is initiated within an organization, there should be some visible improvement to the data. This is definitely wrong. Moreover, the data quality manager is often viewed as having responsibility for some data quality improvement action without necessarily having either the knowledge or authority to make it happen, and the team has no idea where to begin. This is the result of not bringing in the proper expertise to help get the program off the ground.
- Not accounting for organizational culture changes. No technology in the world will eliminate data quality problems without understanding how people’s behavior allows the introduction of information flaws in the first place. Without the cooperation of upstream systems owners, data warehousing managers are often helpless to control the quality of incoming data. Stricter data quality needs at the data warehouse demand resource allocation by upstream managers.
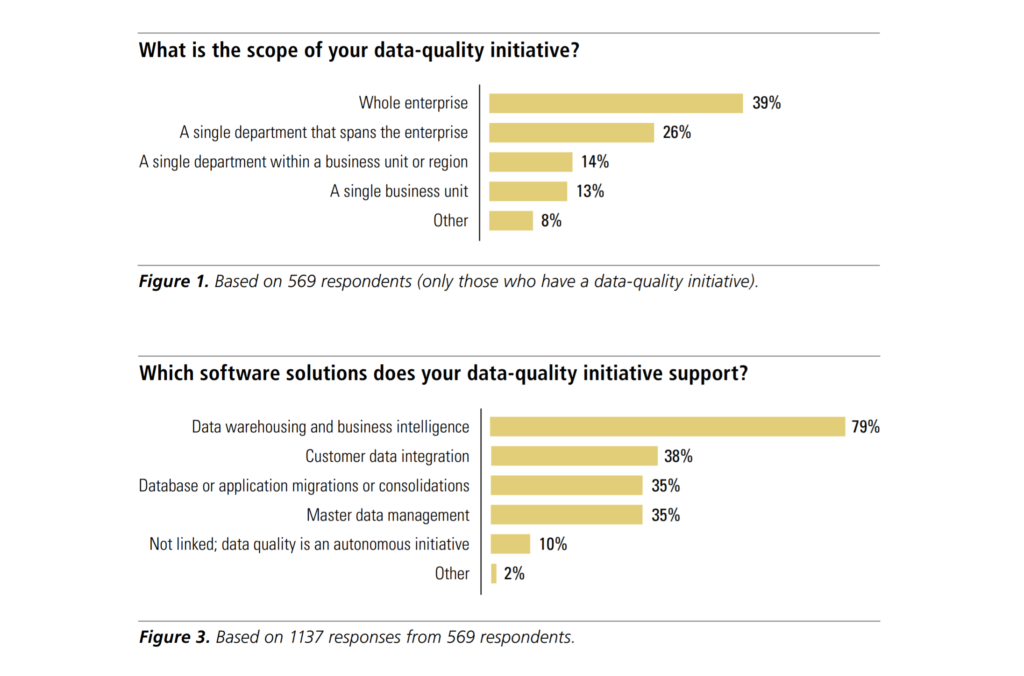
What to do
Here are some of the solutions on how to avoid data quality management mistakes, as provided by David Loshin, an expert in information management:
- Exploit the advisory role of data quality teams and use internal procedures to attach responsibility and accountability for data quality improvement to the existing information management authority.
- Don’t forget training in the use of policies and procedures—especially in the use of acquired tools.
- Hire professionals with experience in managing data quality projects and programs from the start. These individuals will be able to identify opportunities for tactical successes that together contribute to the strategic success of the program.
- Engage external experts to help jump-start the improvement process. This will reassure your team that your problems are not unique and will allow you to learn from others’ best practices.
With proper data governance programs, MDM, and these recommendations, the quality of your data may eventually fit your business requirements.
Further reading
- Poor Data Quality Can Have Long-Term Effects
- Gartner: Companies Lose Millions Due to Poor Data Quality
- Neglecting the Quality of Data Leads to Failed CRM Projects