MDM Longs for Data Quality and Solid Grounds
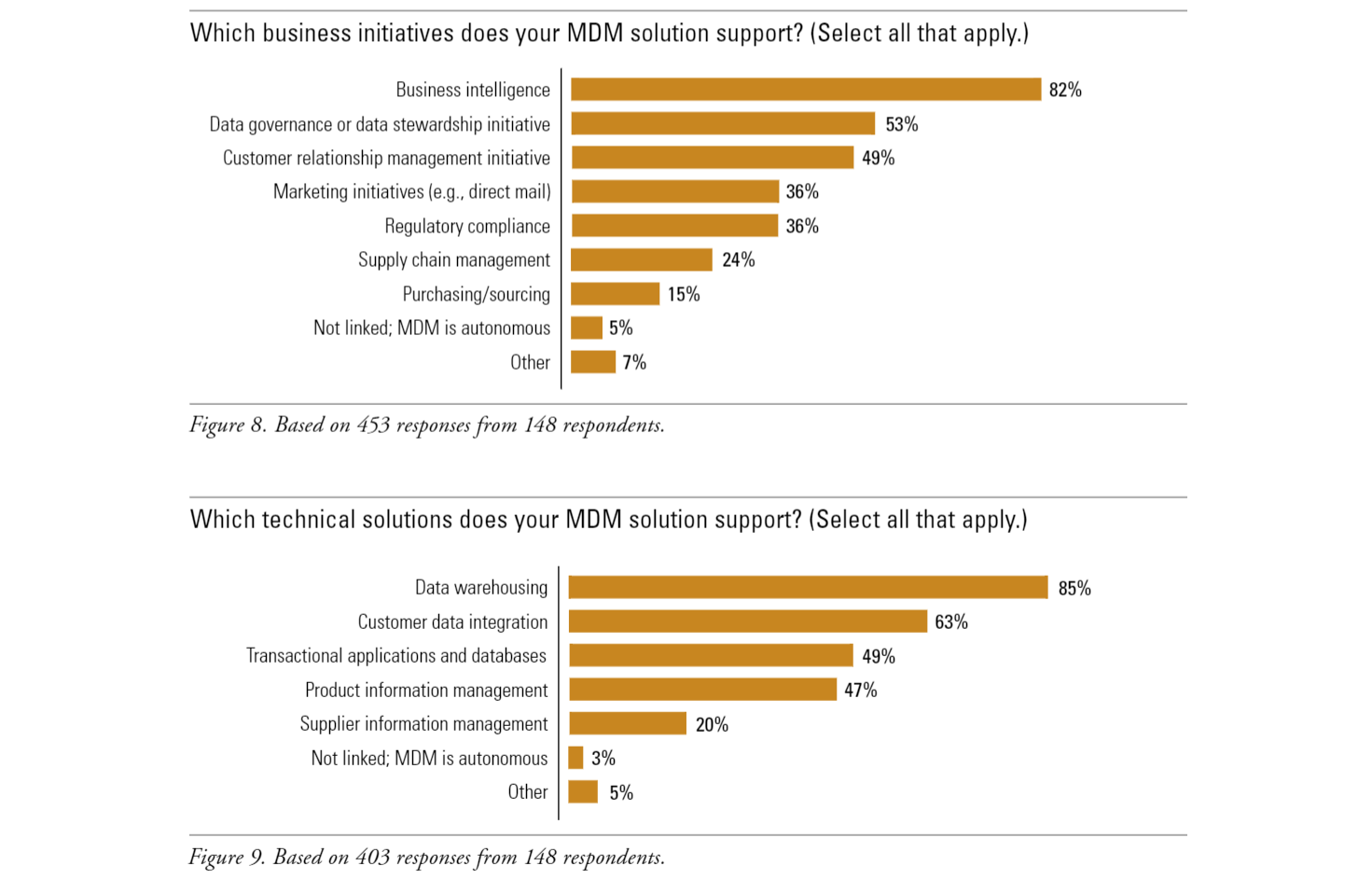
MDM in brief
The objective of Master Data Management is to provide and maintain a consistent view of a company’s main business entities. Many MDM applications concentrate on handling customer data, because it improves sales, marketing, and other business-critical operations.
A unified, consistent view of dispersed information can be created by adopting various data integration techniques and technologies. In addition to creating a data warehouse and utilizing ETL and data quality tools, other related initiatives can be applied, as well:
- • Data consolidation enables to capture data from multiple sources and integrate it into a single data warehouse.
• Data federation provides a single virtual view of source data files.
• Data propagation ensures the distribution of data from one location to another.
It is quite common for MDM applications to use a hybrid approach that involves several data integration initiatives. So, what’s going on in MDM today?
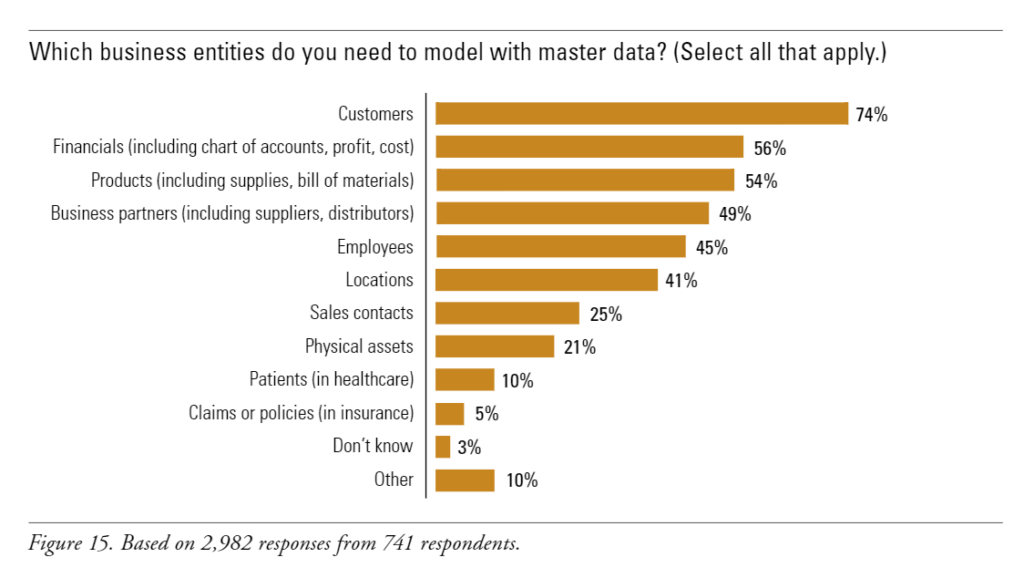
MDM trends
Some time ago, Jill Dyché singled out the current master data management trends for SearchDataManagement.com:
1. The use of data quality tools.
That is, establishing data quality standards and following through with them.
2. Using a common platform for multiple data subject areas.
Yep, the switch to a single platform that would unify your multiple applications and be convenient is taking place. To go further, this is the switch to on-demand applications from your desktop software.
3. Building solid business cases for MDM that transcend the “feeds and speeds” conversation and pitch bona-fide business value of MDM. Take case management in state government—whereby the state can track an individual despite multiple identities and addresses—thereby more quickly targeting food stamp fraud and saving millions in taxpayer dollars.
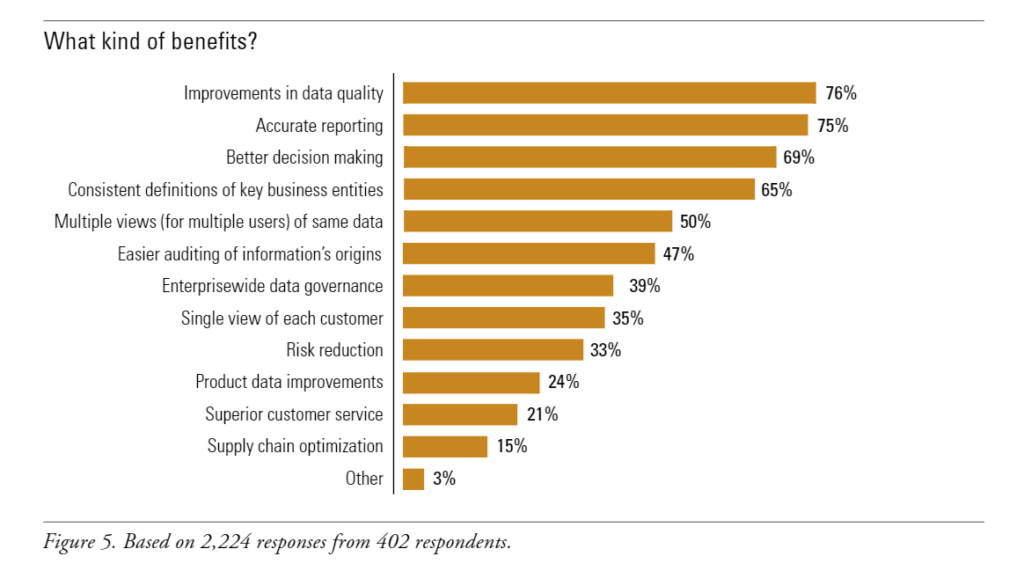
According to Philip Russom of TDWI, though MDM has existed in isolated environments before, “companies now practice it in more silos and with more connections.” Comparing this to the adoption of other related technologies, Philip notes that “the general trend in MDM is toward broader and better integrated enterprise scope.”
For more on the state of MDM, check out his comprehensive report titled “Master Data Management: Consensus-Driven Data Definitions for Cross-Application Consistency” (2006). Indeed, worth reading!
How to improve data quality as part of MDM?
So, data quality management and MDM are two key factors of enterprise information management today. They are interrelated, since without data quality management (DQM), MDM is simply a pile of the data storage. On top of that, DQM cannot bring ROI to the organization without successful MDM. Actually, data quality management plays a role of a building block of an MDM hub, as quality and accurate data is a key to the success of an MDM program.
In-depth analysis of the quality and health of data is a prerequisite of an enterprise-grade MDM program. Here are data quality management steps—suggested by Sanjay Kumar on Information Management—which are needed to support an agile MDM program:
- Identify and qualify the master data and its sources. The definition of master data may be different for different business units. The first step involves identifying and qualifying master data for each business unit in the organization
- Identify and define the global and local data elements. More than one system may store/generate the same master information. Additionally, there could also be a global version as well as local versions of the master data. Perform detailed analysis to understand the commonalities and differences between local, global and global-local attributes of data elements.
- Identify the data elements that require data cleansing and correction. At this stage, the data elements supporting the MDM hub that require data cleansing and correction have to be identified. Communication with the stakeholders is necessary so that as part of the MDM initiative, data quality will be injected into these selected data elements on an organization-wide basis.
- Perform data discovery and analysis. Data collected from source applications needs to be analyzed to understand the sufficiency, accuracy, consistency and redundancy issues associated with data sets. Analyze source data from both business and technical perspectives.
- Define the strategy for initial and incremental data quality management. A well-defined strategy should be in place to support initial and incremental data cleansing for the MDM hub. Asynchronous data cleansing using the batch processes can be adopted for initial data cleansing. Industry-standard ETL and DQM commercial off-the-shelf tools should be used for initial data cleansing. The incremental data cleansing will be supported using synchronous/real-time data cleansing.
- Monitor and manage the data quality of the MDM hub. Continuous data vigilance is required to maintain up-to-date and quality data in an MDM hub. Data quality needs to be analyzed on a periodic basis to identify the trends associated with the data and its impact over the organization MDM program.
In fact, data quality management is the foundation for an effective and successful MDM implementation. A well-defined strategy improves the success probability of an MDM program. Organization should embark a data discovery and analysis phase to understand the health, quality and origin of the master data.
Further reading
- Data Quality: Upstream or Downstream?
- Solving the Problems Associated with “Dirty” Data
- Poor Data Quality Can Have Long-Term Effects