Implementing a Business Rules–Based Approach to Data Quality
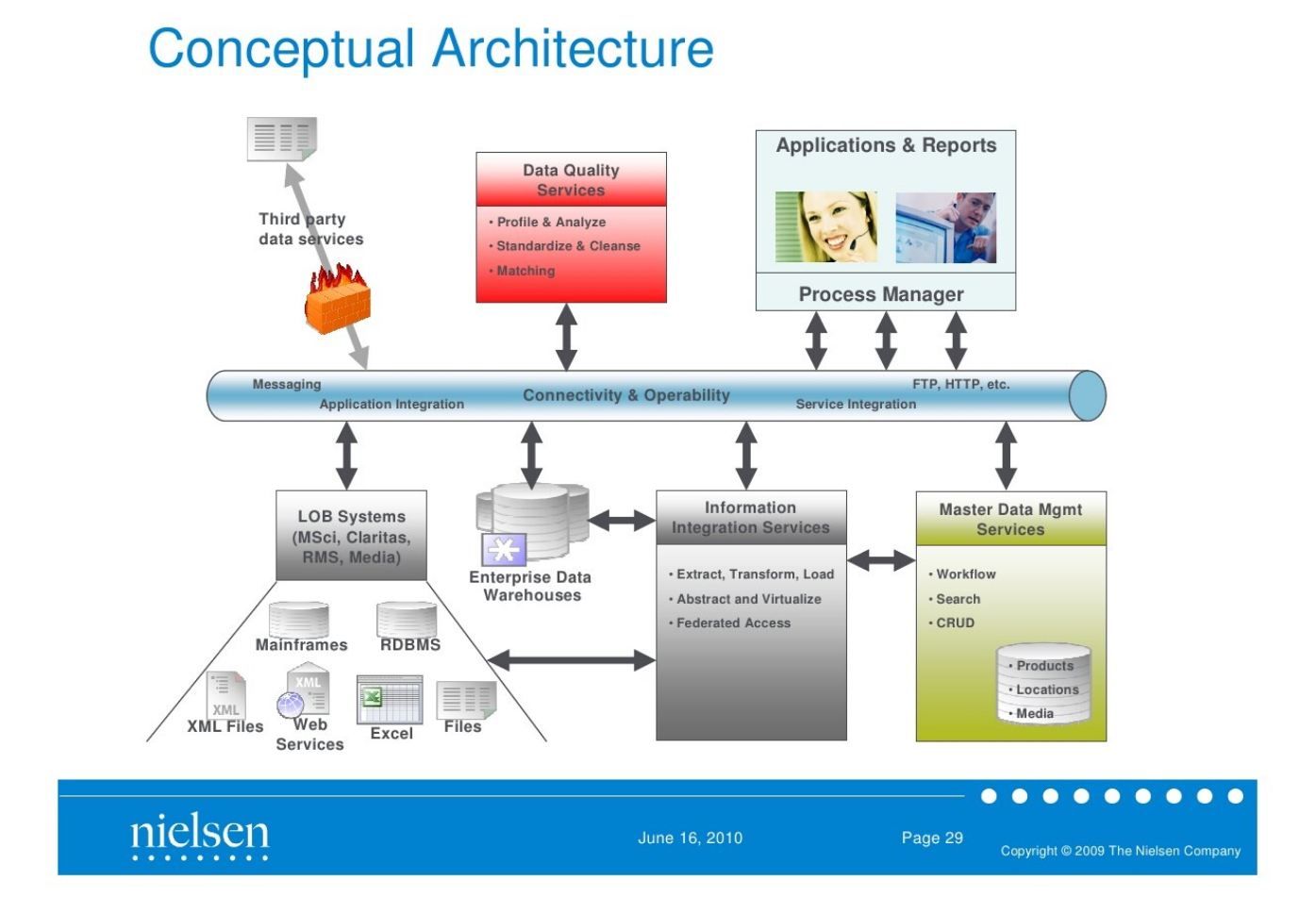
Quality metrics and thresholds
Data integration experts consider information quality the main attribute for business users. Not only does the user need information to be delivered on time, but he or she also wants this information to be of a certain quality. Thus, data quality criteria are required to make this happen.
At the early stages of an information management initiative, technical data quality criteria, such as metrics and thresholds, are usually defined. These data quality parameters are business-independent, and contemporary data integration technologies can automatically evaluate them. These criteria may include:
- Data types
- Data domain compliance (domains refer to a set of allowable values—for structured data, this can be a list of values, such as postal codes, a range of values between 1 and 100, etc.)
- Statistical features of a data set (maximum value, minimum value, population distributions)
- Referential relationships
However, is it enough to ensure data governance? Certainly, not.
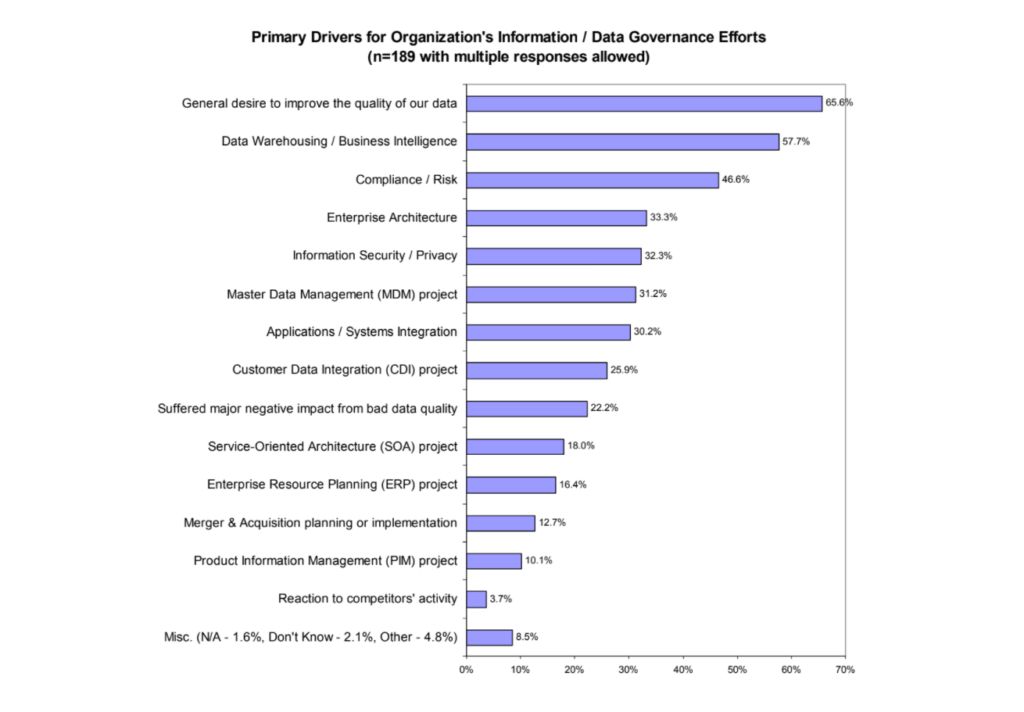
Business rules
To make the most out of an information management initiative for a coporate end user, other measures of data quality should involve business rules compliance. For example, a mobile operator may establish as a business rule the number of months an account has a positive credit balance. All accounts with more than two months of negative balance are considered invalid. These criteria cannot be automatically evaluated solely by a data integration system—business rules should be programmed.
This approach can help to automate the decisions the company makes in its day-to-day operations. This also can be used to audit data for compliance with both external and internal regulations and policies. (Read the best practices for incorporating business rules into data governance / stewardship efforts in this article from The Enterprise Information Management Institute.)
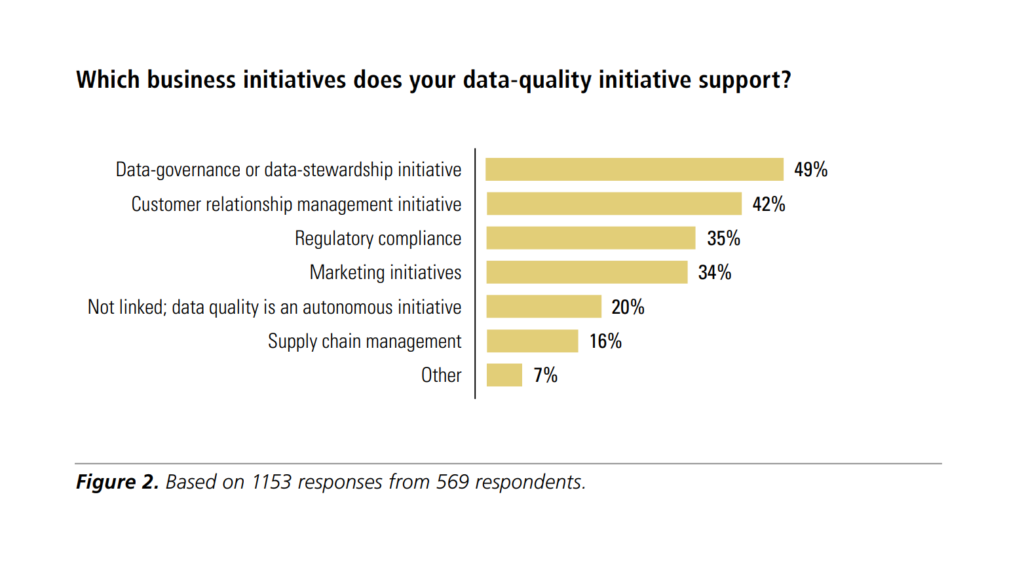
So, before acquiring a data quality solution, an organization should establish a set of business rules that later have to be transformed into code. Still, there is a number of chalenges, such as flexibility and limitations of this transformation, as well as other low-level issues known with data integration—e.g., processing data formats when aggregating / consolidating several sources of information.
Challenging data formats
ETL tools should be able to find a standard way of handling a large variety of source and target data formats. This functionality is needed to implement comprehensive business rules within your data integration solution. Here’s how you can optimize an ETL tool to avoid issues with multiple data formats:
- Make data normalization rules descriptive, don’t hide them in procedural code blocks. Enable business people to specify rules in terms that make sense to them.
- Set clear identification and reporting rules to detect the impact of data format changes, as well as maintenance rules, when a change occurs.
- Rules should be expressed in terms of context-independent concepts that can easily be referred to by business people.
- Do not express business rules in physical field names, as it will require normalization functions to be created for each new source data format.
Some ETL tools force developers into a variety of steps and complex procedures for accommodating the variation in source and target data formats. They lack the features to clearly express business rules (or comply with them), and therefore can hardly be leveraged by nontechnical users.
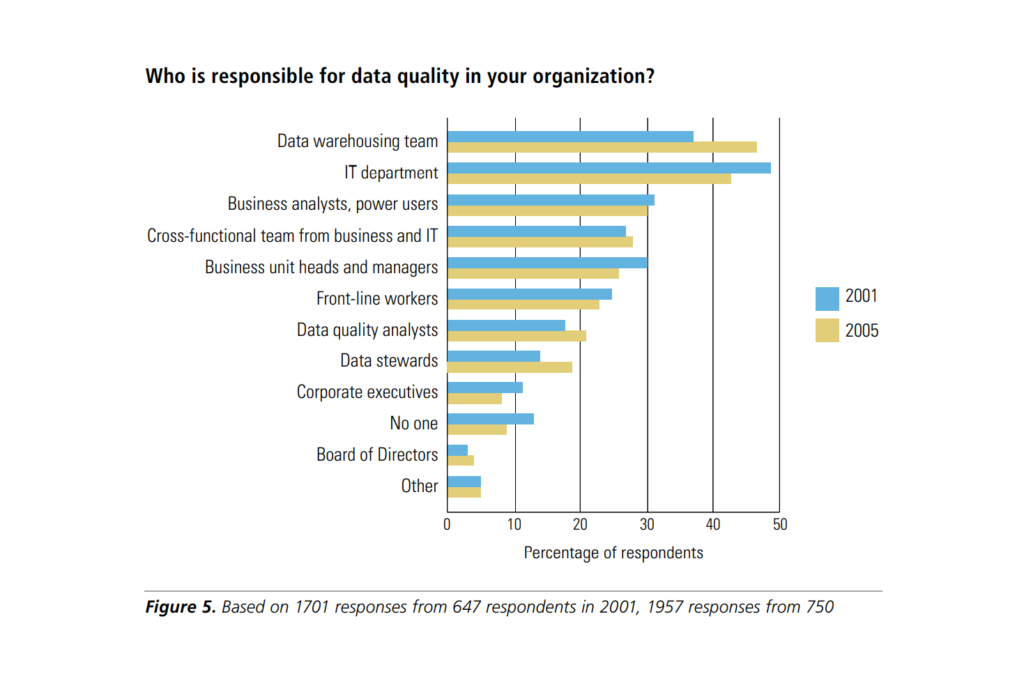
For more best practices on implementing business rules for improving data quality, check out these best practices and 10 mistakes to avoid from David Loshin.
Related slides
Further reading
- The Reasons for Poor Data Quality
- Solving the Problems Associated with “Dirty” Data
- MDM Longs for Data Quality and Solid Grounds