Top 7 Challenges of Product Traceability in Manufacturing Supply Chain
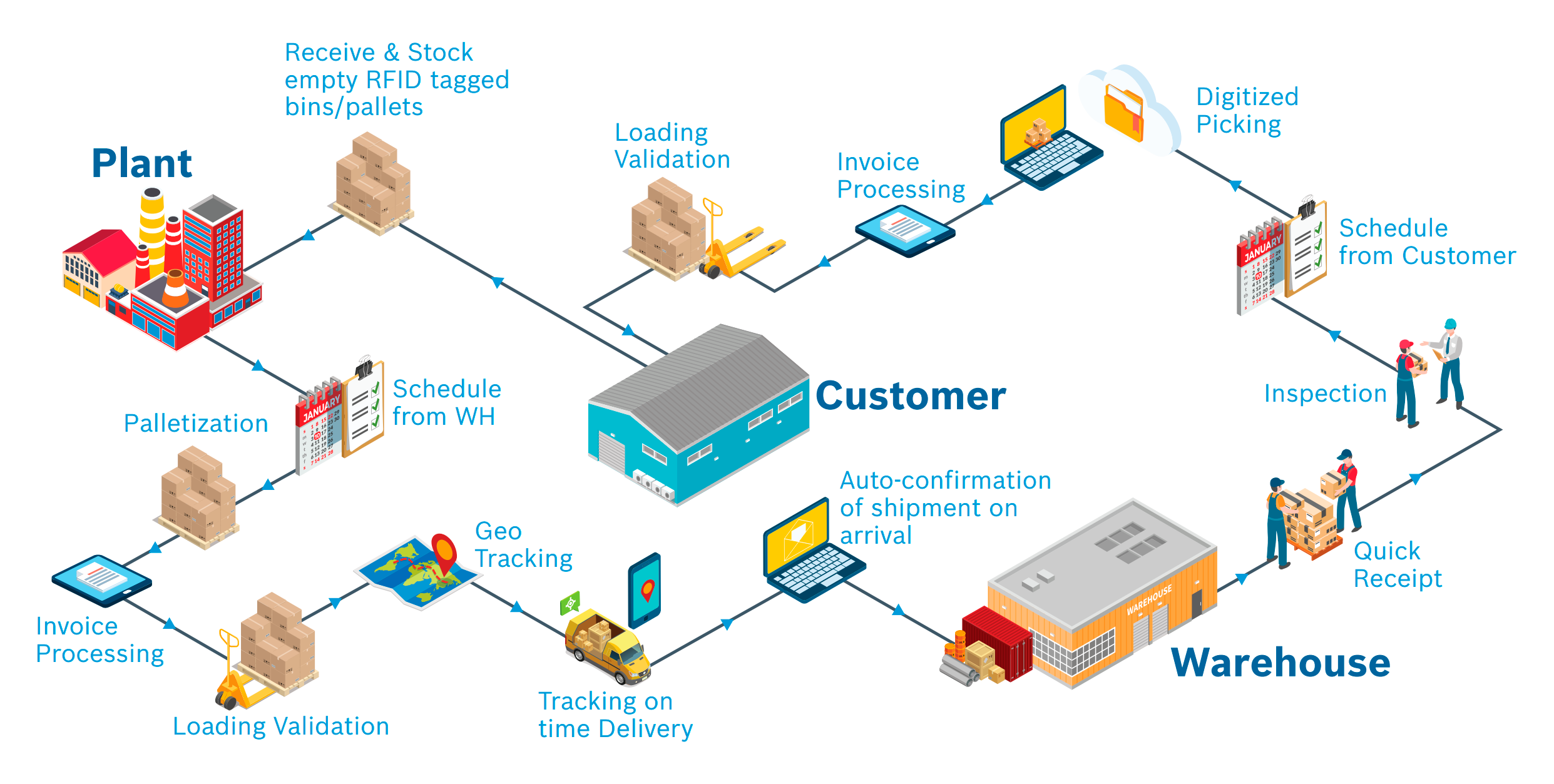
Supply chain traceability is as important as ever
The digital supply chain market is being disrupted rapidly. On November 14, 2022, Microsoft introduced its Supply Chain Platform enabling organizations such as Daimler to improve product traceability. Two weeks later, Amazon announced AWS Supply Chain as a response to Microsoft’s release. Even though Amazon’s platform is currently available in preview, and an enterprise-ready version will be expected later, why is the competition so high and time-sensitive?
You, probably, remember the Suez Canal obstruction in March 2021, when a 400-meter vessel got stuck and single-handedly disrupted the global supply chain. The incident happened in the middle of the COVID pandemic, when most of the industries were facing delays and shortages. After analyzing the situation, Bain & Company suggested that enterprises with enabled product/materials tracking could minimize losses, being able to find alternative suppliers.
However, a study by Deloitte published in October 2022 highlights the scale of manufacturers’ visibility issues. Only 13% of respondents could “fully map their supply chain networks,” with 72% having partial or limited visibility beyond their “tier-2” network. Another recent report from EY confirmed that only 37% of respondents have experienced “increasing visibility” in 2022.
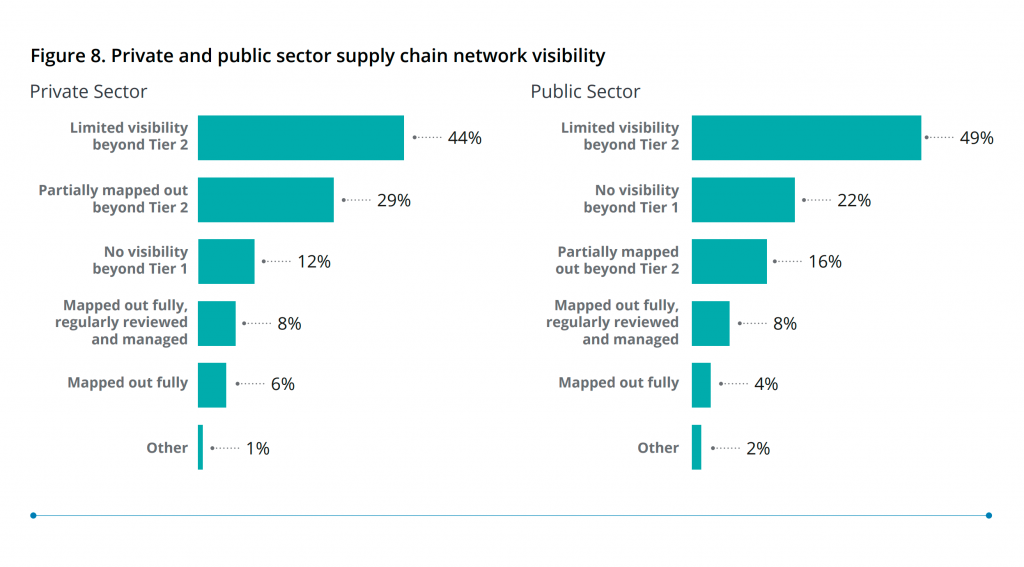
So, why do companies pursue supply chain traceability and visibility? For instance, for the suppliers in the life sciences industry, Everest Group discovered the following needs:
- minimizing product loss and theft
- recall procedures for expired or noncompliant goods
- fighting fraud and counterfeit products
- compliance (e.g., see the Drug Supply Chain Security Act)
- sustainability requirements (to track packaging waste / emissions and identify eco-friendly routes)
In addition, the transparent flow of goods helps to design just-in-time (or lean) manufacturing, reducing warehousing expenses. It’s no surprise that increasing efficiency, reskilling the workforce, and improving visibility are the top priorities for supply chain executives, according to an EY report (January 2023).
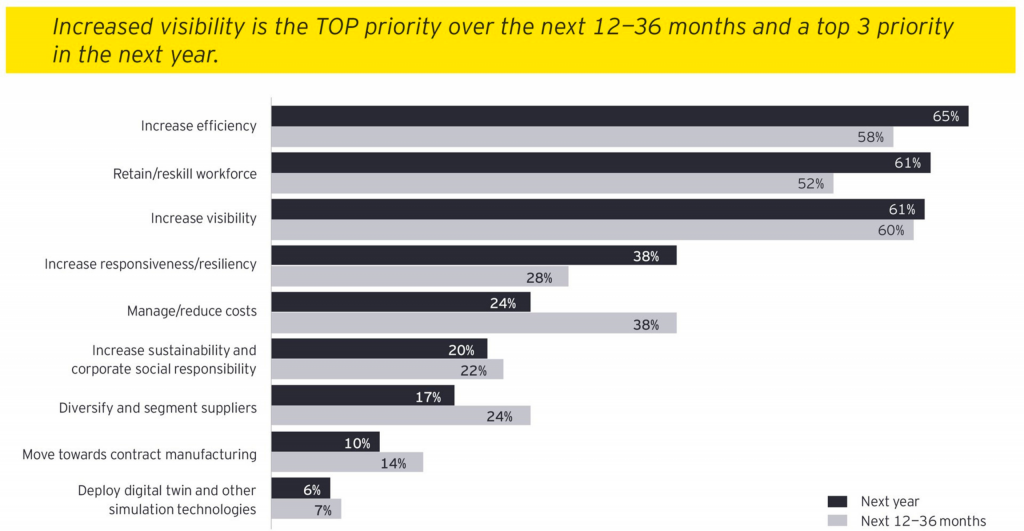
Though funding and the lack of a clear strategy are obvious bottlenecks for implementing supply chain traceability, the process is not simple for those who have started it, too. In the following sections, we’ll cover the major complexities of product tracing implementation and provide potential guidelines to address the issues.
1. Track-and-trace data reliability
The process of identifying raw materials or manufactured products and then monitoring their location or movement is generally called “track and trace.” The Internet of Things (IoT) platforms collect necessary data via smart devices and then transmit it for analysis or reporting. Sensors, GPS trackers, and RFID tags provide location data, being distributed across factories, warehouses, and vehicles.
However, these devices are not 100% perfect and may introduce some fraction of error—due to inaccurate mounting, heating/freezing, aging, or malfunction. On a large scale, with a myriad of trackers, this may bring in a series of unreliable records, leading to concept drift and “dirty data.” The issue escalates in the case of low-cost devices.
Another problem is that barcode readers scan the packaging, but not the product itself. Therefore, scanning the packaging doesn’t guarantee that the sensitive/fragile product (such as ice-cream or wine) has arrived safe. So, the amount of goods tracked can differ from the actual one, with no to little information about the condition of the products.
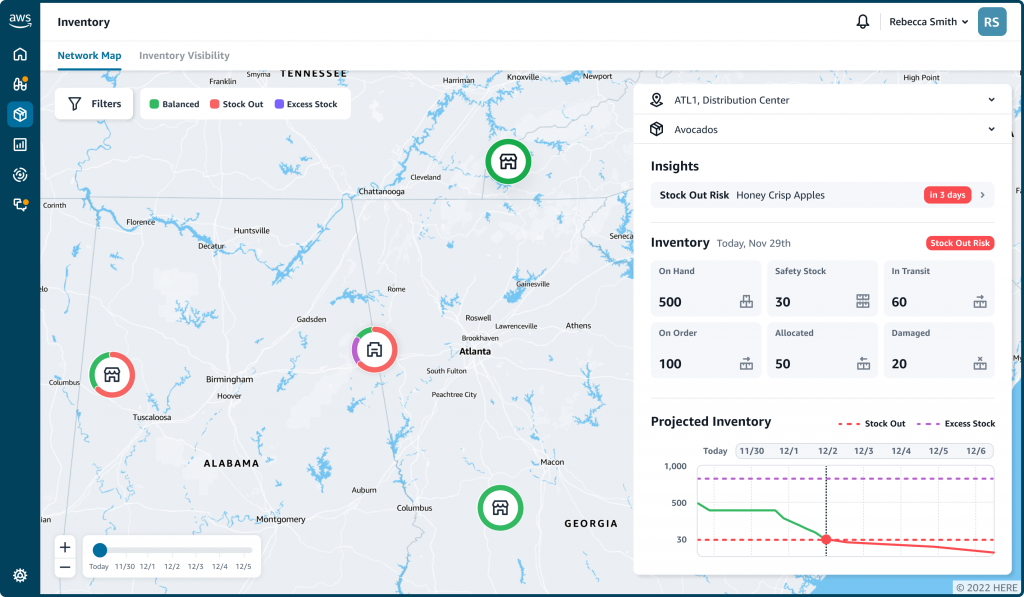
Still, the question is, how many sensors do you need to ensure product visibility? What type of devices should you purchase? How much data to collect to ensure the information is reliable enough? How to enable traceability within factories or other facilities where GPS is unavailable?
Therefore, the costly track-and-trace implementation is not an obvious process and requires thorough planning upfront and designing an ROI-driven strategy. The World Economic Forum (WEF) and Bain & Company recommend focusing on a pilot for specific opportunities that will create immediate value and then expand, amplifying the results.
On a large scale, to ensure the health of IoT equipment, one can rely on a device management platform. This solution performs a variety of supervision tasks remotely—including malfunction detection and firmware updates. (The AWS IoT Device Management and Azure IoT Hub documentation describe similar services available on the two major cloud platforms.) It’s also worth investing into anomaly detection to spot outliers or fraudulent activities, triggering proper actions, such as device maintenance or access denial.
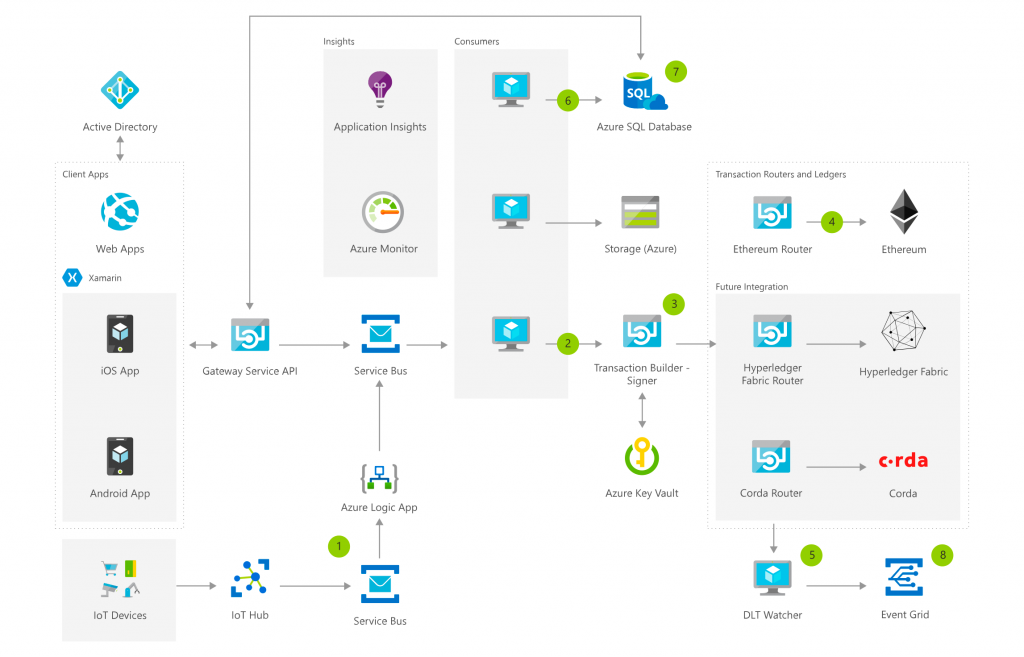
In addition to location data, a supplier may also need to track additional parameters for food, medicine, and other sensitive products. Cold-chain delivery monitoring can verify whether the temperature and humidity were within the limits during shipments, which is essential for dairy products and pharmaceuticals. A viable method to ensure the safe transportation of fragile goods (such as glass) involves installing and utilizing vibration sensors within containers. To increase the reliability of product tracing or double-check weight, a company may consider integrating digital scales and computer vision (see the next section).
Finally, in facilities where GPS fails to work, indoor positioning may also be helpful.
2. Manual operations increase errors, delays, and costs
Many procedures enabling supply chain traceability in manufacturing are performed manually, adding the burden to data reliability. For instance, track-and-trace operations involve scanning thousands of products or parcels with handheld devices, while invoice data is manually entered into corporate systems. This method is labor-intensive, time-consuming, and prone to human error. Such issues can snowball into multiple inaccuracies and delays—resulting in lost or returned products and unreliable forecasts or analytics—which eventually escalates costs.
To streamline product traceability where manual efforts are required, a company should automate as many processes as possible and label all the physical items involved. Barcodes, QR codes, and computer vision can be applied to both goods and pallets used for transportation. To make code scanning more accessible and reduce the costs, distributors might replace professional handheld devices with regular mobile apps.
Additional tools and devices for traceability within factories or warehouses may monitor pick/pack operations to ensure each carton contains the right goods in the correct amount. Microsoft explains that we can find similar mechanisms in conveyor belts with integrated sensors and computer-vision features. Other companies opt for picking carts with built-in scales.
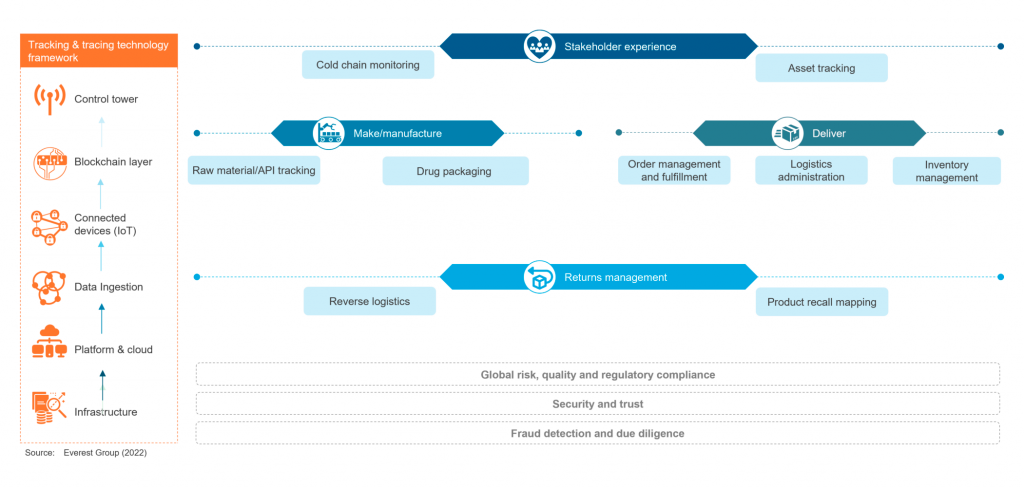
Gartner also predicts that “artificial intelligence (AI) and machine learning (ML) will have the greatest impact on the supply chain industry through 2025.” Some of the supply chain scenarios will include RPA for reducing paperwork (digitizing invoices with object recognition) and automatically processing orders.
3. The huge amount of interconnected data
When implemented, though, traceability software, barcode readers, and IoT sensors generate massive streams of real-time data coming from factories, warehouses, vehicles, etc. The problems with data management start from the very beginning—collection—and escalate as the system operates.
First, supply chain data can be very heterogeneous in terms of type (material volumes, shipment dates, etc.) and source (IoT sensors, ERP systems, etc.). Second, manufacturers may deal with both structured and unstructured data (varying from dates in a delivery schedule to satellite imagery for route optimization). Third, seasonal / holiday spikes in demand contribute to peak data loads that can put a strain on logistics software. Fourth, the correlation between goods / products and spare parts / materials coming from multiple suppliers can be extremely complex.
Therefore, comprehensive data management should be part of your supply chain strategy—covering data quality, metadata management, data warehousing, etc.
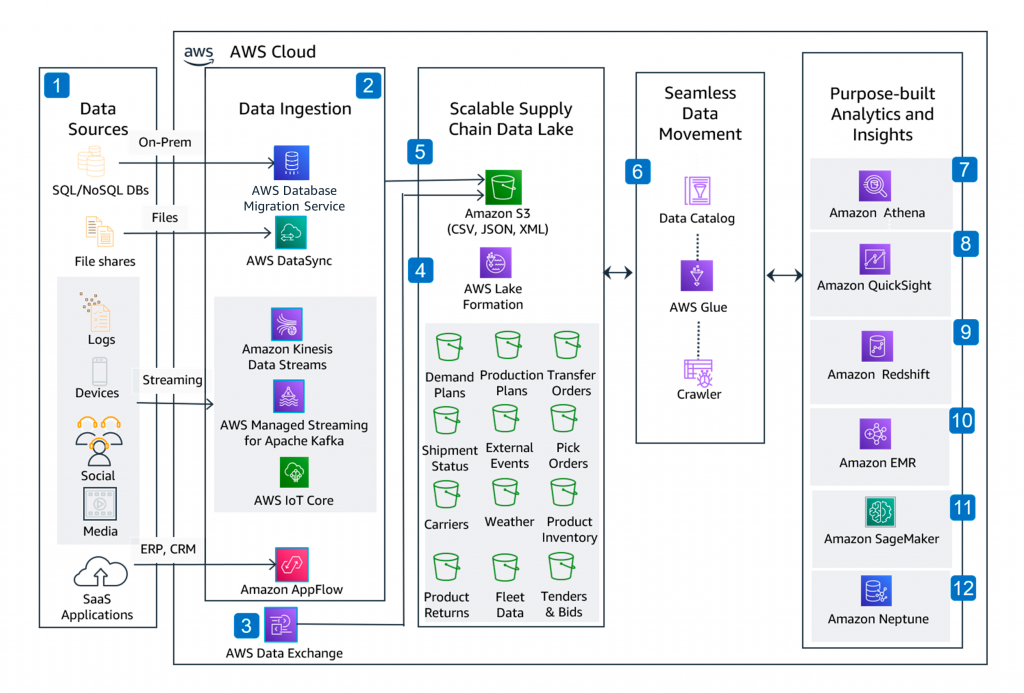
Besides, to collect this abundant information, data lakes can enable companies to ingest and consolidate these inputs in their raw format, with no need for transformations. This tutorial from Amazon explains how to turn a data lake into a centralized repository to keep information for future analysis (including items whose value is still not clear). Another article details how to integrate third-party supply chain systems—such as those for transportation / warehouse / inventory management or freight forwarding—on AWS.
In addition, to address scalability and performance, NoSQL databases and in-memory storages may help. Graph databases can also simplify mapping relationships between products, materials, suppliers, manufacturers, etc.
4. A fragmented picture
Even after collecting sufficient telemetry and ensuring data reliability, companies may still have limited supply chain visibility due to information silos. This happens when data is stored in isolated systems, creating a fragmented view about product movement.
Why does this happen? A typical manufacturing software ecosystem encompasses a multitude of applications that collect and process data covering specific areas of the supply chain. CRM software stores customer data, while ERP systems handle corporate information regarding most business functions or reporting. Manufacturing execution systems (MES), on the other hand, take care of monitoring manufacturing processes, such as the transformation of materials into goods.
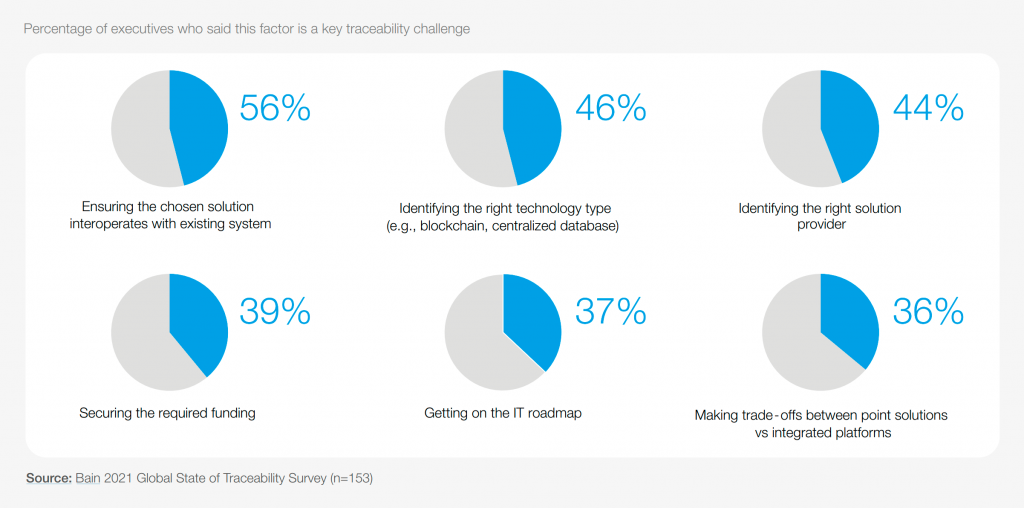
A standalone supply chain traceability system for manufacturing makes the workflow even more complicated. As a result, Bain & Company’s 2021 survey revealed that 56% of executives consider ensuring interoperability the main product traceability challenge. All this calls for data integration—very often, in real time.
Depending on the existing infrastructure, this may require creating an enterprise service bus (ESB), building a centralized middleware, or adopting data sync tools. To minimize fragmentation issues, manufacturers can also go for unified platforms offered by major providers, such as Microsoft, Amazon, or SAP.
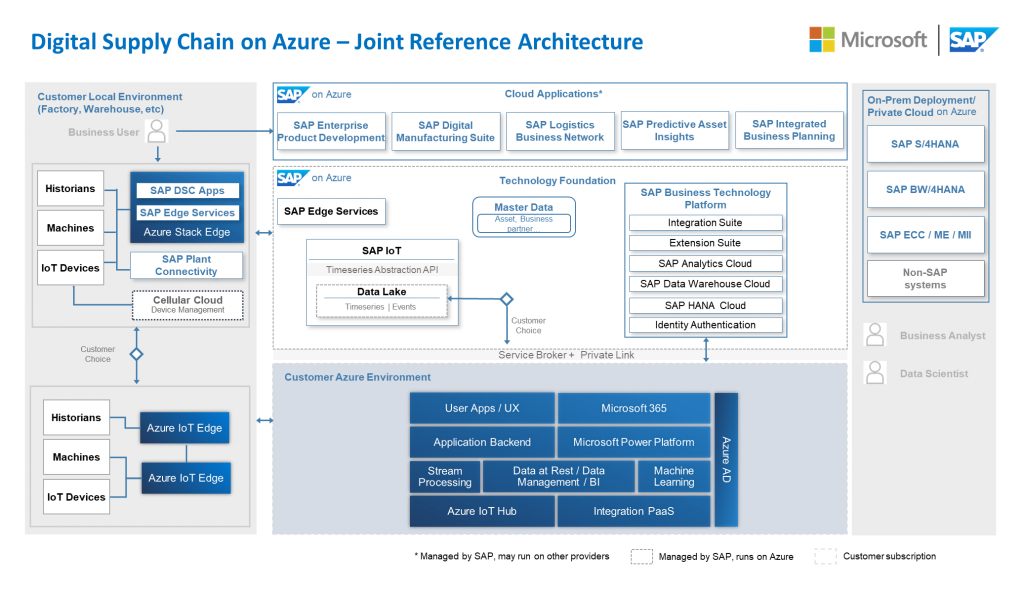
5. Turning data into valuable insights and predictions
As the WEF and Bain & Company reported, value does not come from data per se, but from analytics and predictive modeling. This means processing data from IoT sensors, logistics software or supplier systems, and turning it into actionable insights. For example, data analytics software may examine historical information to identify the reasons behind a production bottleneck, such as delayed delivery of key components. As a result, this solution could provide recommendations on alternative routes or suppliers.
It is also possible to forecast upcoming product demand spikes based on seasonal trends, helping organizations to address the challenges timely. McKinsey estimates (2022) that autonomous supply chain planning based on intelligent predictions and analytics can result in 4% revenue growth and a 10% cost reduction. Predictive maintenance is another step toward making value out of data. By monitoring asset conditions (e.g., vehicles or sensors), one can detect anomalies and carry out preventive repairs.
However, data analytics for decision-making introduces its own requirements, according to Hitachi. First, analytical solutions should be fuelled with relevant data. Second, this data should be properly cleansed and prepared—most likely, unified or transformed. Third, it may have limited integrability / interoperability, as we wrote above. As a result, analytical software can be quite expensive to build and maintain, not to mention the requirements for proper expertise (in BI, ETL, OLAP, data science, etc.).
Predictions should also be based on accurate, reliable, and consistent data. Otherwise, a data model created with ML to simulate scenarios, including demand or shipment arrival time, will be unstable, leading to incorrect forecasts. Still, ML requires a huge computing power and time. ML algorithms go through a complex training process, involving large data sets, and building a predictive model can take months.
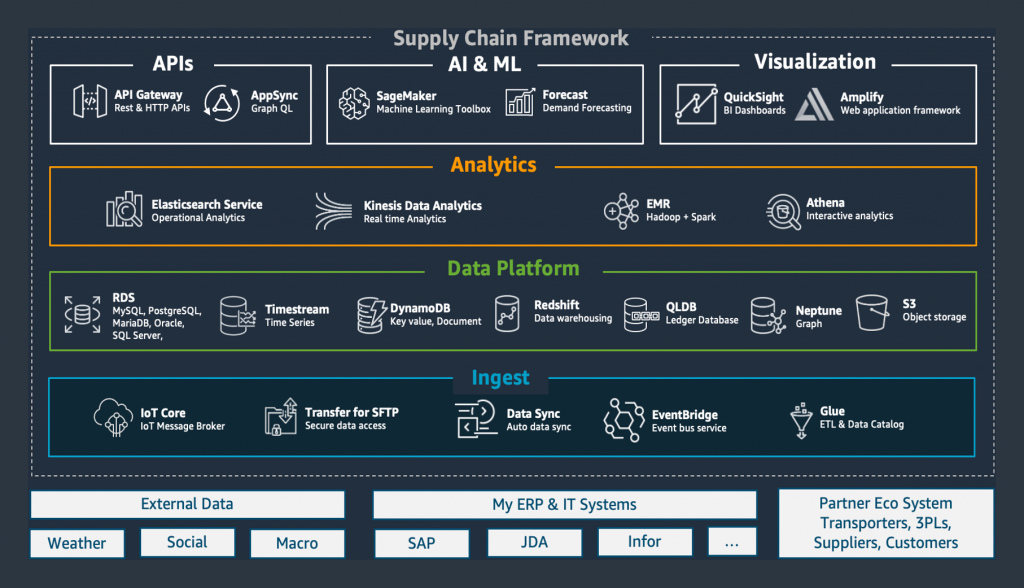
The WEF and Bain & Company suggest defining data requirements based on the corporate strategy and mapping information across supply chain systems. This will enable managers to identify essential data for specific use cases while avoiding information that can’t be turned into actionable insights.
All this brings in another reason to properly handle the issues described in the previous sections—including inaccurate sensor readings and information silos. To prepare and unify data for reporting and analysis, organizations may need to set up necessary ETL pipelines, data warehousing, cleansing, labeling, etc.
To accelerate the delivery of data analytics, manufacturers may opt for existing platforms, such as Microsoft Power BI, Azure Synapse Analytics, Amazon Redshift, etc. Сloud vendors also offer integrated ML services to facilitate predictions, including Amazon Forecast and SageMaker. These platforms provide built-in algorithms, pretrained ML models, and scalable computing resources.
It’s also worth mentioning a recent trend to combine data analytics systems into broader suites known as supply chain control towers. As described by Gartner, these act as unified hubs, providing real-time visibility and in-depth analysis. Control towers can also drive automation via artificial intelligence and machine learning.
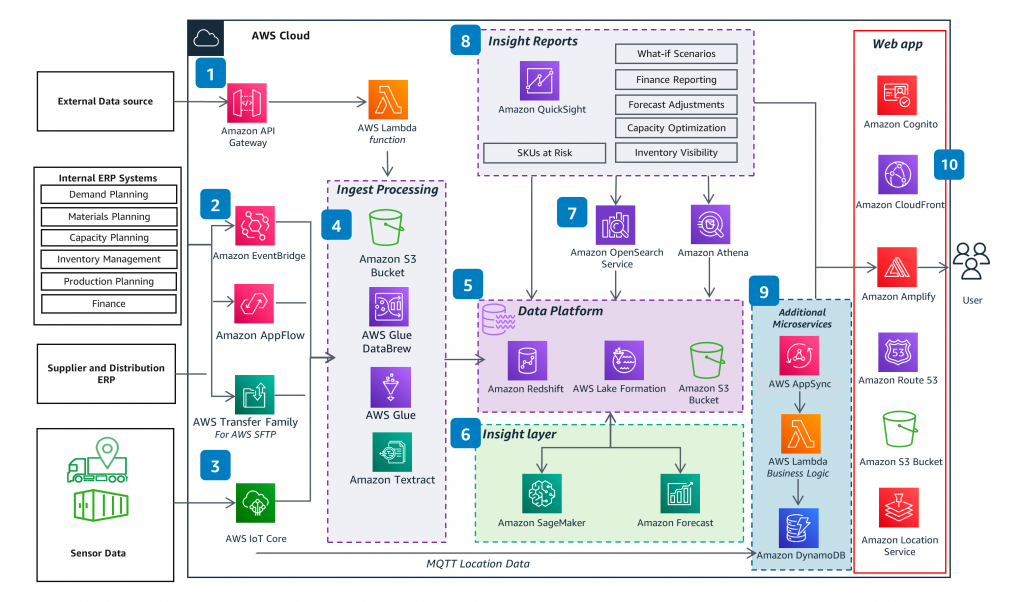
Other powerful initiatives manufacturers can utilize for analytical purposes are supply chains’ digital twins. These involve virtual representations based on IoT device data, portraying the manufacturing and logistics network in real time. A Gartner report (June 2022) claims that digital twins promote situational awareness and end-to-end decision-making across organizations and partner ecosystems.
6. Transparent, security-first data sharing
So, as we can see, visibility requires quality, real-time data. At the same time, manufacturing and logistics involves multiple operators focusing on specific procedures of the supply chain. This means that end-to-end product traceability requires cooperation among partners (suppliers, producers, logistics companies, insurers, etc.), including data sharing.
In particular, the WEF and Bain & Company reported that 64% of executives considered collaboration very or extremely important to access traceability-enabling data. Gartner confirms this, naming ecosystem collaboration among the top 8 supply chain technology themes in 2022. The analyst calls for adopting collaborative environments and mapping tools to visualize real-time multi-tier connections across people, data, machines, systems, and processes.
However, organizations may have a hard time gaining supply chain data from each other. Partners may be reluctant to share information to maintain a competitive edge, or simply lack the technology to handle data properly and safely.
Organizations can use shared standards and networks that feature unique product and location identifiers (e.g., the Global Trade Item Number and Global Location Number). Other standards, including EPCIS, focus on creating a shared view of business-relevant processes and objects (trade items, logistic units, etc.). Furthermore, networks of data pools like the GDSN help trading partners to exchange product data in real time.
Another option to address data sharing complexities is blockchain. According to McKinsey, blockchain shows great promise for improving supply chain traceability, decreasing risks, and optimizing transaction costs. On a blockchain, information is verifiable and unmodifiable, ensuring irreversible transactions (shipments, payments, loans, etc.) and safer data sharing. Various multinational companies are already turning to this technology for product traceability in manufacturing—such as Walmart, Alibaba, and Boeing.
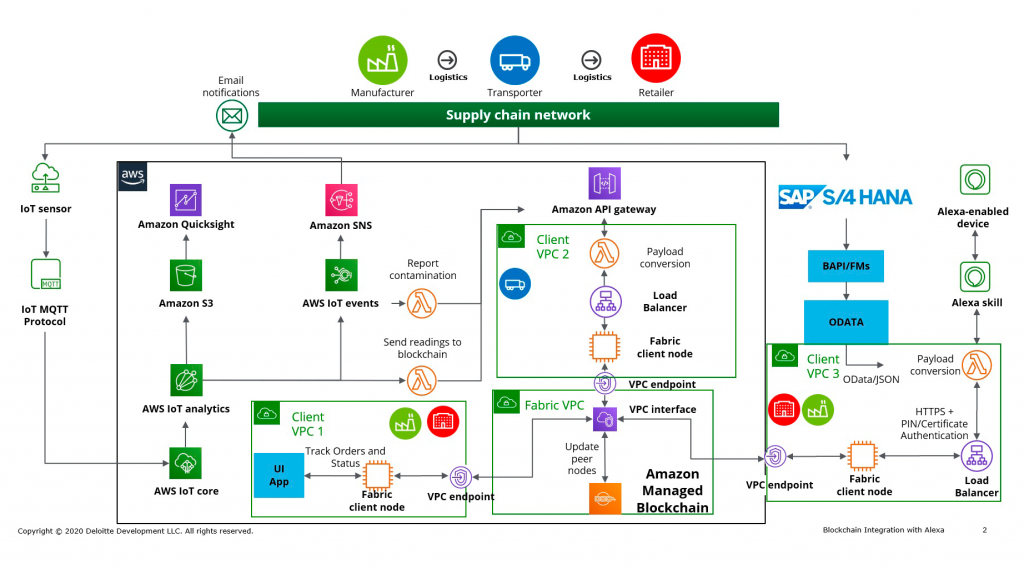
In a similar manner, an automotive manufacturer partnered with us to develop a blockchain platform for tracking vehicles’ spare parts. With this solution, the customer reduced the efforts to perform end-to-end supply processes by 6.4x, cut warranty expenses by 7%, and shortened dispute resolution time from 3 weeks to 2 days.
7. Protecting product data across the supply chain
Still, the amount of shareholders, software, and IoT devices involved in a supply chain result in multiple points of vulnerability. This way, organizations may face hacking, unauthorized access to corporate networks, and data leaks.
For example, hackers may affect routers with a DNS poisoning attack or target IoT devices with trojans to redirect traffic toward nonstandard endpoints. Other threats come from packet analyzers, which intercept traffic on wireless networks. Compromised assets might include product quantities and components, serial numbers, destination of goods, and other data that can identify a customer.
This means that protecting a product traceability system for manufacturing from such threats and ensuring data privacy should be considered a top priority. Deloitte reported (September 2022) that 88% of manufacturers surveyed consider supply chain ecosystems as sources of cybersecurity threats and related issues.
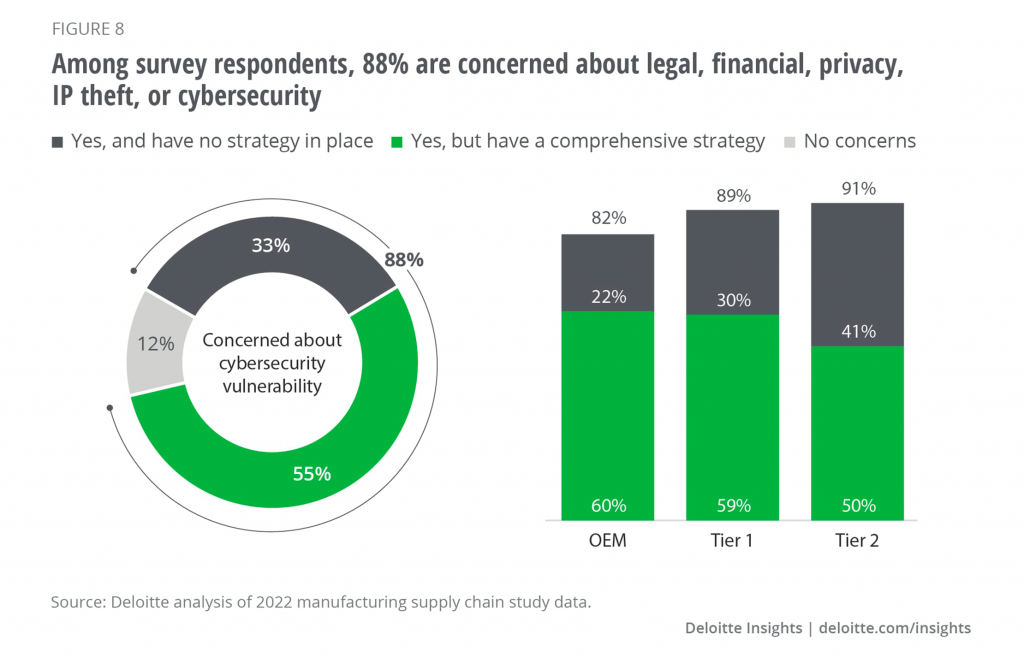
Among the measures to safeguard IoT systems in a supply chain, IBM enumerates encrypted data exchange, multifactor authentication, and session breaks. Microsoft suggests implementing various IoT device authentication options, including X.509 certificates and symmetric keys.
In its turn, Gartner brings forward the concept of a “security mesh” to address the vulnerability points of the supply chain. A security mesh aims at building an integrated ecosystem of tools protecting access to data, assets, and participants in the supply chain. With this granular access control, it is possible to early detect malicious activity and act accordingly, implement consistent security policies across the board, etc.
To safeguard supply chain traceability solutions based on the IoT, Gartner also suggests implementing a zero-trust approach to identity access management. Microsoft backs up this idea by stating that a zero-trust security model grants a more granular control over access to data. This is essential, taking into account the amount of agents in the supply chain.
Key enablers of the supply chain visibility
So, product traceability in manufacturing brings multiple challenges—both organizational and technical in nature. To make the process easier, the WEF and Bain & Company recommend that the implementation should rely on five interconnected “enablers”:
- an operating model adapted to cross-functional strategies
- a partner ecosystem
- integrated approach to data
- a solid technology architecture
- compliance
Small, incremental milestones should be combined with a scaling plan across use cases with maximum value. By relying on a trusted partner, companies can streamline the adoption of necessary technologies, addressing supply chain traceability in manufacturing.
Further reading
- IoT in Transportation and Logistics: Use Cases, Challenges, and Best Practices
- Scaling Industrial IoT in Manufacturing: Challenges and Guidelines
- A Close Look at Everledger—How Blockchain Secures Luxury Goods